Photo by Viktor Hesse on Unsplash
Introduction
In the intricate world of oil trading, where fortunes are made and lost in the blink of an eye, the ability to decipher market signals is paramount. Oil trading algorithms have become indispensable tools for traders seeking to navigate the complexities of this volatile market. These algorithms employ sophisticated techniques to analyze vast amounts of data and extract valuable insights, allowing traders to make informed decisions and stay ahead of the curve. In this article, we will delve deep into the inner workings of oil trading algorithms, exploring how they decode market signals to drive profitable trading strategies. Bitcore Momentum serves as a cutting-edge solution for traders looking to harness the power of algorithmic trading in the oil market.
Fundamentals of Oil Market Signals
Market signals serve as the lifeblood of oil trading algorithms, providing vital information about supply, demand, and price dynamics. These signals can take various forms, ranging from price movements and volume changes to geopolitical events and economic indicators. Understanding and interpreting these signals is essential for traders looking to capitalize on opportunities and mitigate risks in the oil market.
Role of Big Data in Oil Trading
At the heart of oil trading algorithms lies the utilization of big data. These algorithms leverage vast amounts of data from diverse sources, including satellite imagery, government reports, and social media, to gather comprehensive market intelligence. The quality and quantity of data play a crucial role in algorithmic analysis, as algorithms rely on accurate and timely information to generate reliable trading signals. By harnessing the power of big data, oil trading algorithms can identify emerging trends, assess market sentiment, and anticipate price movements with greater precision.
Key Components of Oil Trading Algorithms
Oil trading algorithms employ a combination of technical and fundamental analysis techniques to interpret market signals. Technical analysis involves the study of historical price data and trading volumes to identify patterns and trends. Common technical indicators used by algorithms include moving averages, stochastic oscillators, and relative strength index (RSI). In contrast, fundamental analysis focuses on factors such as supply and demand dynamics, geopolitical developments, and macroeconomic indicators to assess the underlying value of oil assets. By integrating both technical and fundamental analysis, oil trading algorithms can generate more robust trading signals and improve decision-making accuracy.
Machine Learning and AI in Signal Analysis
In recent years, machine learning and artificial intelligence (AI) have revolutionized the field of signal analysis in oil trading. These advanced technologies enable algorithms to learn from past data, adapt to changing market conditions, and improve performance over time. Machine learning algorithms can analyze vast datasets to identify complex patterns and correlations that may not be apparent to human traders. By leveraging AI-driven models, oil trading algorithms can enhance predictive accuracy, optimize trading strategies, and minimize risk exposure.
Algorithmic Strategies for Signal Interpretation
Oil trading algorithms employ a variety of strategies to interpret market signals and execute trading decisions. These strategies range from simple trend-following techniques to more sophisticated arbitrage and momentum-based approaches. Trend-following algorithms aim to capitalize on sustained price movements by identifying and riding market trends. Arbitrage algorithms exploit price discrepancies between different markets or instruments to generate profits with minimal risk. Momentum-based algorithms seek to capitalize on short-term price fluctuations by entering and exiting trades quickly based on momentum indicators. By deploying a combination of these strategies, oil trading algorithms can adapt to changing market conditions and optimize trading performance.
Case Studies and Examples
Real-world examples illustrate the effectiveness of oil trading algorithms in decoding market signals and driving profitable trading strategies. For instance, a study conducted by researchers at a leading investment bank found that oil trading algorithms outperformed human traders in terms of both profitability and risk management. These algorithms were able to identify lucrative trading opportunities and execute trades with precision, resulting in superior returns compared to traditional trading methods. Similarly, another study by a prominent hedge fund demonstrated the effectiveness of machine learning algorithms in predicting oil price movements based on historical data and market sentiment analysis. These case studies highlight the transformative impact of algorithmic trading on the oil market and underscore the importance of data-driven decision-making in modern trading environments.
Future Trends in Algorithmic Signal Analysis
Looking ahead, the future of algorithmic signal analysis in oil trading holds great promise. Emerging technologies such as blockchain, quantum computing, and natural language processing (NLP) are poised to revolutionize the way market signals are analyzed and interpreted. Blockchain technology, for example, offers decentralized and transparent data management solutions that could enhance data integrity and security in algorithmic trading systems. Quantum computing holds the potential to accelerate complex calculations and simulations, enabling algorithms to process larger datasets and uncover deeper insights into market dynamics. Meanwhile, NLP algorithms can extract valuable information from unstructured data sources such as news articles, social media posts, and analyst reports, providing traders with a more comprehensive understanding of market sentiment and trends. As these technologies continue to evolve, we can expect to see further advancements in algorithmic signal analysis, leading to more sophisticated trading strategies and improved performance in the oil market.
Conclusion
In conclusion, oil trading algorithms play a pivotal role in decoding market signals and driving successful trading strategies in the oil market. By leveraging big data, machine learning, and AI-driven models, these algorithms can analyze vast amounts of information, identify profitable trading opportunities, and mitigate risk exposure. However, it is essential to recognize that algorithmic trading is not without its challenges, and success ultimately depends on the careful design, implementation, and monitoring of trading algorithms. As technology continues to evolve and new innovations emerge, we can expect to see further advancements in algorithmic signal analysis, reshaping the landscape of oil trading and empowering traders with powerful tools to navigate the complexities of the market.
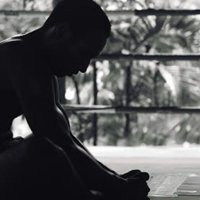
Daniel J. Morgan is the founder of Invidiata Magazine, a premier publication showcasing luxury living, arts, and culture. With a passion for excellence, Daniel has established the magazine as a beacon of sophistication and refinement, captivating discerning audiences worldwide.